A while back Amy Grace Wells, one of the editors of UX Booth, pitched me an article idea:
What do you think of an article that explains, compares, and contrasts the ideas of learnability, searchability, discoverability, and findability and how each can affect UX?
I mulled it over, since… how do you explain how all these things work together to create a user experience that’s usable in just one article? This is the bread and butter of modern experience design here. People make their entire careers focusing on just one of these concepts. And here I am, asked to write the definitive UX Booth how-to article on all of it.
So I sat with it for a few months, wrote some notes, and waited for inspiration.
And then 14 inches (36cm) of inspiration landed on my front lawn. Seattle had its snowiest two week period in a century. We had four storms that paralyzed a city that already shuts down for a single snowflake.
In between two of the biggest storms, I managed to shovel the car out of the driveway and make it to the supermarket.
I hate grocery shopping. I have to put all my searching skills to work in this loud, disjointed experience where they seem to move all the product locations capriciously. But we were low on food that wasn’t “dried beans of an unknown vintage” or “leftovers that look like they were frozen during the last ice age,” and I have a family that doesn’t find any of those things any more appetizing than I do.
A Walk-through on Searchability
My wife texted me a list that included:
- Bananas
- Potato chips
- Maybe some frozen things (if we were going to be trapped indoors another four days, might as well load up on easy dinners)
Bananas. That’s easy. Walk in the door, take a right, go to the produce section of the market. The bananas are on a stand in the middle, in various shades of unripe green and too ripe yellow.
Bananas are searchable. I know, roughly, where to look for them. They’re also findable: They are obviously placed, easily identifiable. All I have to do is pick the right quantity (how many bananas?) and ripeness level (will we eat them today or three days from now?) and I’m done.
The store’s architecture helps with searchability — it makes it very clear where the produce is. It’s spotlighted, all the produce is out for easy inspection, the greens are with the greens, the fruit with the fruit.
Every grocery store in North America has a similar produce section with similar piles of bananas. A European or Asian grocery store also has an area set aside for fresh produce — it’s not going to be stacked on a shelf next to the flour or the snacks. Though there are local variations and quirks, it is only the rare store where you can’t find the bananas. Just find the produce section.
Learnability Is Repeatable
This pattern to grocery store produce sections makes them learnable. Patterns like this are valuable in the design of any system. Without repeatability, users do not trust systems to produce the same quality of results every time. Google’s searches are repeatable because, despite all the SEO and SEM we may throw at it, it will (almost) always return a reliable set of results whose pattern is recognizable as the same one we’ve seen the other 10,000 times we’ve searched on the web.
The searchability of a produce section dovetails with the findability of its products. Where searchability relies on the system and the patterns created, findability makes those products, well, findable. I know exactly what a banana looks like, and the prominence of the banana display will help me match my banana pattern and make my decision.
A system designed for findability keeps those things in mind. It focuses on showing the right results — with the right cues (visual, auditory, physical) — for any search. If it’s something people tend to look for more, it’s more prominently displayed; for example, you’ll see fruit like apples and peaches out in full display, while rutabagas and radishes will be packed in next to the greens. The success of the system relies on the user’s ability to identify the exact thing they came looking for. It’s not buried in an FAQ somewhere or lost down a twisty click path.
Bananas now in hand, we’re on to potato chips. And here I’m in a small quandary: What kind of potato chips? There are a lot of brands and sizes… not to mention an incredible number of flavors. (The sheer number of BBQ flavor chips at the local market is a sight to behold. You want Backyard BBQ? Or Honey Mustard BBQ? Or maybe Korean BBQ? They got ‘em. And some 15 others.)
I have to use my knowledge of what my family will eat. For example, sour cream and onion is always a hit, while pepperoncini — yes, that’s a real flavor — I can keep all to myself. (And sometimes I buy them only for that reason.)
I also know brands matter here. Lay’s sour cream and onion are light and thin and favored over the thicker kettle chips, while my kid’s love of Tim’s Cascade Original trumps all other offerings.
This is where discoverability would come into play. Discoverability is different from findability — instead of looking for one specific thing (a banana), you’re looking at all your available options to pick the one(s) best for you. You have specific needs, but they are only about finding A Thing, not The Thing.
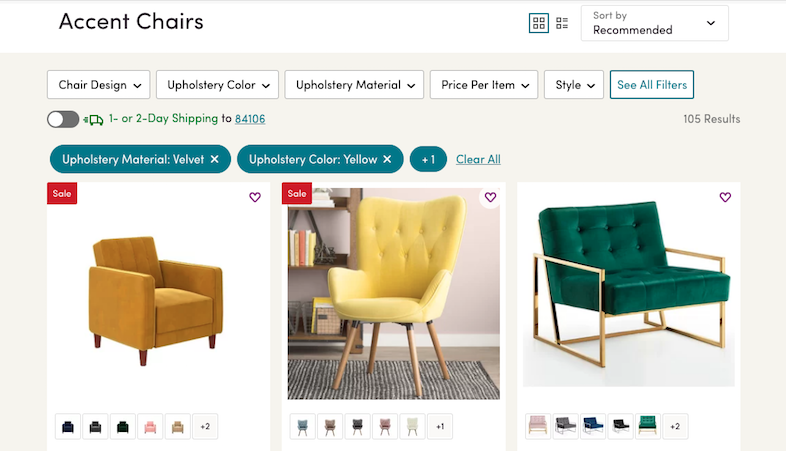
When we refer to the experience of discoverability in search we usually look to faceted search. Faceted search gives the user the ability to change search results based on categories and filters to reduce the options displayed. Faceting has been around since the days of the card catalog, but it was only when information systems could instantly cross-reference all the categories and tags that modern faceted search became possible.
Discoverability on the Web
A modern e-commerce site can do all this. Consider the time I needed to replace my clothes dryer. There were some key things I was looking for: gas instead of electric, a particular size to fit the space, a signal to say when drying was complete, something in white to match the washing machine. At the same time, I didn’t need a wi-fi enabled dryer; I was brand agnostic; and I really didn’t care if it had the modern steam drying tech or not. The store websites I looked at all gave me the ability to control the result set, giving me a smaller number of options to browse.
The physical space, though, is different. You can’t just physically rearrange the bags of potato chips based on your particular mental hierarchy of salty fried goodness. And a store can’t do that, either—not without immense cost. Stores have an information architecture they prefer, and they ask you to map your own mental model to theirs. Chips are arranged by brand, by primary ingredient (potato? corn? pita?). You then have to visually sort through them and identify the kind of sour cream and onion chip you want.
Sometimes, though, it creates taxonomical problems. Take the Frito, a fried corn chip made of a sort of masa. One would think it would be in the same section as tortilla chips, which are also made from masa and fried. But, they’re (almost always) not. Is this right?
Or take maple syrup. Some stores put it near pancake mix, showing a correct (North American) contextual pattern — pancakes (and waffles) go with maple syrup. Others, though, put the syrup with sugar and the pancake mix with the cake mix, often aisles apart. Which one is the right pattern?
Good discoverability can only exist with a solid system for searchability that is contextually optimized for findability.
Dark Patterns
I’m running into another problem with the potato chips, though: The store moved the aisle. Learnability is great in the banana business, but not so much something favored in the potato chip concern.
Here we’re encountering the dark patterns around discoverability. When we talk about discoverability on the web, we usually end up talking about SEO and optimizing what the org wants its users to see. They want their site near the top of the search results, and they’ll do anything to get there, even to the detriment of their users.
When you are in a grocery store, this experience is typified by the endcap — the shelves at the end of the aisle that showcase promoted or sale products. The shopper passing by can be drawn by things they didn’t know they needed. It’s perfect for the impulse buy, which helps keep the store in business, but it is also a distraction.
Moving the potato chip aisle is another dark pattern. It breaks your ability to easily find, forcing you to rely on your foraging instincts. You’ll have to roam the store looking for the chips, and along the way, you’ll have to scan all the aisles and any signposting pointing to the right place. The fundamental searchability of the store remains intact, but the findability is being subordinated by discoverability. This may frustrate you, but like endcaps, this dark pattern helps a store make more money.
Culminating in Searchability
The frozen food aisle is where all these ideas come together. Freezers are hard to move, so stores can’t hack your mental map of the store as easily as, say, shifting the potato chips across the building. Thus, learnability is built in to the architecture of the store. They can break learnability by rearranging the items in the frozen section, though. And they often do.
Searchability thus relies heavily on signposting — this is where the frozen pizzas are, this is where the ice cream is. The search is more landmark-based than driven by a well-ordered taxonomy of ice-cold edibles.
When you walk into a store with a vague request like “maybe some frozen things,” you are bringing indecisiveness into a system that relies on your indecisiveness to slow you down and make you spend more.
Luckily, this is where Marcia Bates and “berrypicking” steps in to help.
Berrypicking – A Lesson in Web Search Patterns
Bates proposed a search retrieval system in 1989 that relied not on the classical, transactional “match the document to the need through query” model of information retrieval, but instead on a model that she described as “berrypicking”:
“In real-life searches in manual sources, end users may begin with just one feature of a broader topic, or just one relevant reference, and move through a variety of sources. Each new piece of information they encounter gives them new ideas and directions to follow and, consequently, a new conception of the query. At each stage, they are not just modifying the search terms used in order to get a better match for a single query. Rather the query itself (as well as the search terms used) is continually shifting, in part or whole. This type of search is here called an evolving search.”
“Furthermore, at each stage, with each different conception of the query, the user may identify useful information and references. In other words, the query is satisfied not by a single final retrieved set, but by a series of selections of individual references and bits of information at each stage of the ever-modifying search. A bit-at-a-time retrieval of this sort is here called berrypicking. This term is used by analogy to picking huckleberries or blueberries in the forest. The berries are scattered on the bushes; they do not come in bunches. One must pick them one at a time. One could do berrypicking of information without the search need itself changing (evolving), but in this article, the attention is given to searches that combine both of these features.”
30 years ago, Bates described not just the web as we know it today — an assortment of rabbit holes and Wikipedia trails that lead to real discovery — but my exact dilemma in trying to figure out what to do with the request for frozen things. Instead of treating this as a straight search for food, I’ll pick off what best fits the requirements, knowing full well I’m not looking for anything in particular. Frozen pizza? Yeah, they’ll eat that. That makes me think of… a lasagna? A casserole? And thus I am able to forage across the freezers, throw items in my basket, and stock up enough to get us through the next storm.
In berrypicking, we see modern discoverability take root. Search is no longer about singular transactions of matching content to person, but about the complex web of search queries, the decisions we make after each query, and constantly modifying the wandering search to pick off each ripe berry of information. Marcia Bates called this “browsing,” and indeed, robust browsing requires robust discoverability, and influencing that discoverability is paramount to the modern web.
Search engine marketing (SEM) is the most common way we see organizations try to influence this browsing and berrypicking. Showing what was searched or purchased before and offering suggestions based on them is another. (This, of course, needs to work appropriately for the context — Amazon for years offered vacuum cleaner purchasers suggestions of… more vacuum cleaners to purchase. Eventually someone realized that people who buy vacuum cleaners don’t need more vacuums; they need vacuum cleaner bags and replacement parts.)
And thus, I completed my shopping list and got out before the next snowstorm hit. My goals were met. No one in my family went without bananas, potato chips, or frozen pizzas. A grocery store isn’t the web, though. It’s a physical environment that has distinct disadvantages — it can’t change contexts on the fly, can’t provide facets and filtering to winnow your choices, and can’t remember what you came in looking for last time.
An online grocer, on the other hand, can do all these things. In Amazon Fresh or Safeway.com, we find the principles we found in the physical supermarket built out on the web. Searchability through the same search patterns and tools we expect from a generation of e-commerce sites. Learnability from following those patterns. Findability through filters and facets on brands, departments, and other areas. Discoverability through suggestions, product catalogs, and history.
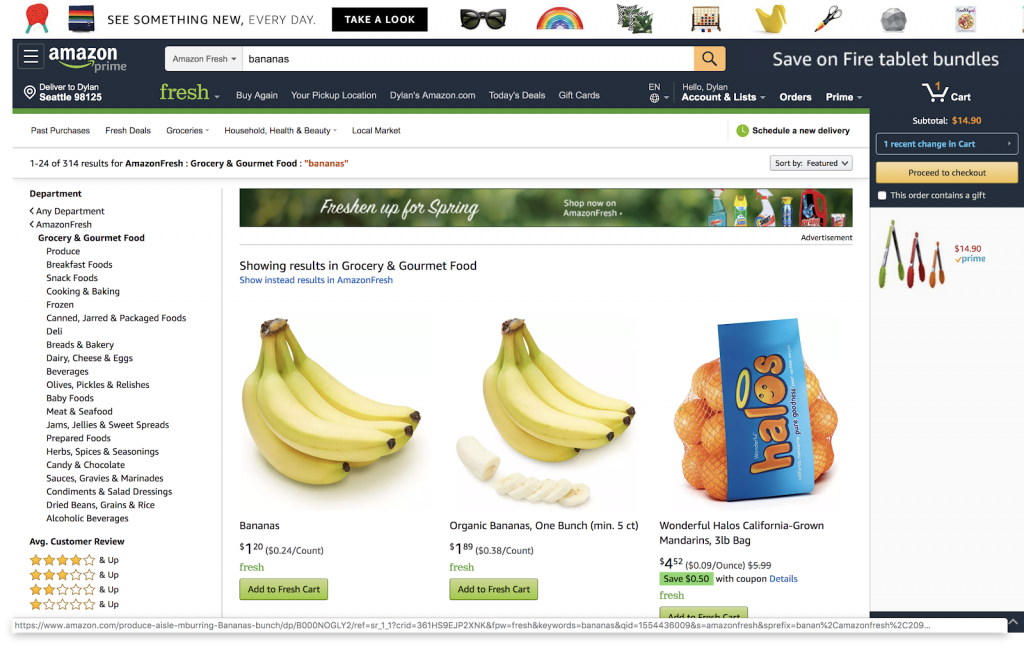
It’s not just e-commerce, of course. Highly searchable systems are the backbone of information catalogs of all type, from libraries to media catalogs. When these systems are learnable you see the emergence of shorthand search language, like Google’s search operators. Findability surfaces in any system with filters and facets, like the aforementioned media catalogs. Discoverability drives any recommendation system, including Netflix’s immense algorithm.
When everything is working well, we can find what we’re looking for by browsing and winnowing, find again using the same patterns, and utilize our berrypicking talents to find the things we’re looking for across hundreds of thousands of possibilities. When it’s not working well, the experience is so disjointed that people start asking for suggestions, for filtering, for search results that are predictable from common search queries.
As experience designers, we need to keep all four in mind regardless of the environment our users roam in:physical, virtual, or — in augmented reality — both. We are working with patterns that date from the earliest times of information retrieval, even patterns born of trying to determine whether something was edible or not. In following the principles of learnability, searchability, discoverability, and findability, we find meaningful, useful experiences for the people that use the things we design.
Information architecture is an often misunderstood job title. Are they designers? developers? managers? All of the above? In this article we'll discuss what information architecture is, why it's related to usability, and what are the common tools/programs used in information architecture.