At the UX Poland Conference, presenter Jeff Parks, said: “Without research, businesses cannot make informed decisions.” By analyzing data, entrepreneurs and UX professionals get information that will help them develop more efficient and profitable products and services.
Contextual analytics is a great way to get the most out of big chunks of data. Facebook and Twitter, for example, are excellent sources of data, but detecting patterns in order to make sense of all that available information is incredibly time-consuming and complicated. Going a step further, once we make sense of the data, we need to analyze it in order to create valuable insights, and that relies on having a specific context in mind – otherwise we end up with nothing but generalized conclusions.
In short, applying contextual analytics can help emphasize the individuality of the consumer and his or her behavior.
What is big data?
To start with, big data is called “big” for a reason – we’re talking terabytes and terabytes of information flowing into companies every day. Regular data becomes “big data” when it is large enough that it cannot be easily processed using conventional methods. With this volume, spreadsheets are useless; they lack flexibility and scalability. However, once processed, that big data is very valuable. With the context provided by analytics, big data can highlight key metrics, allowing UX professionals to create tailored solutions for their users.
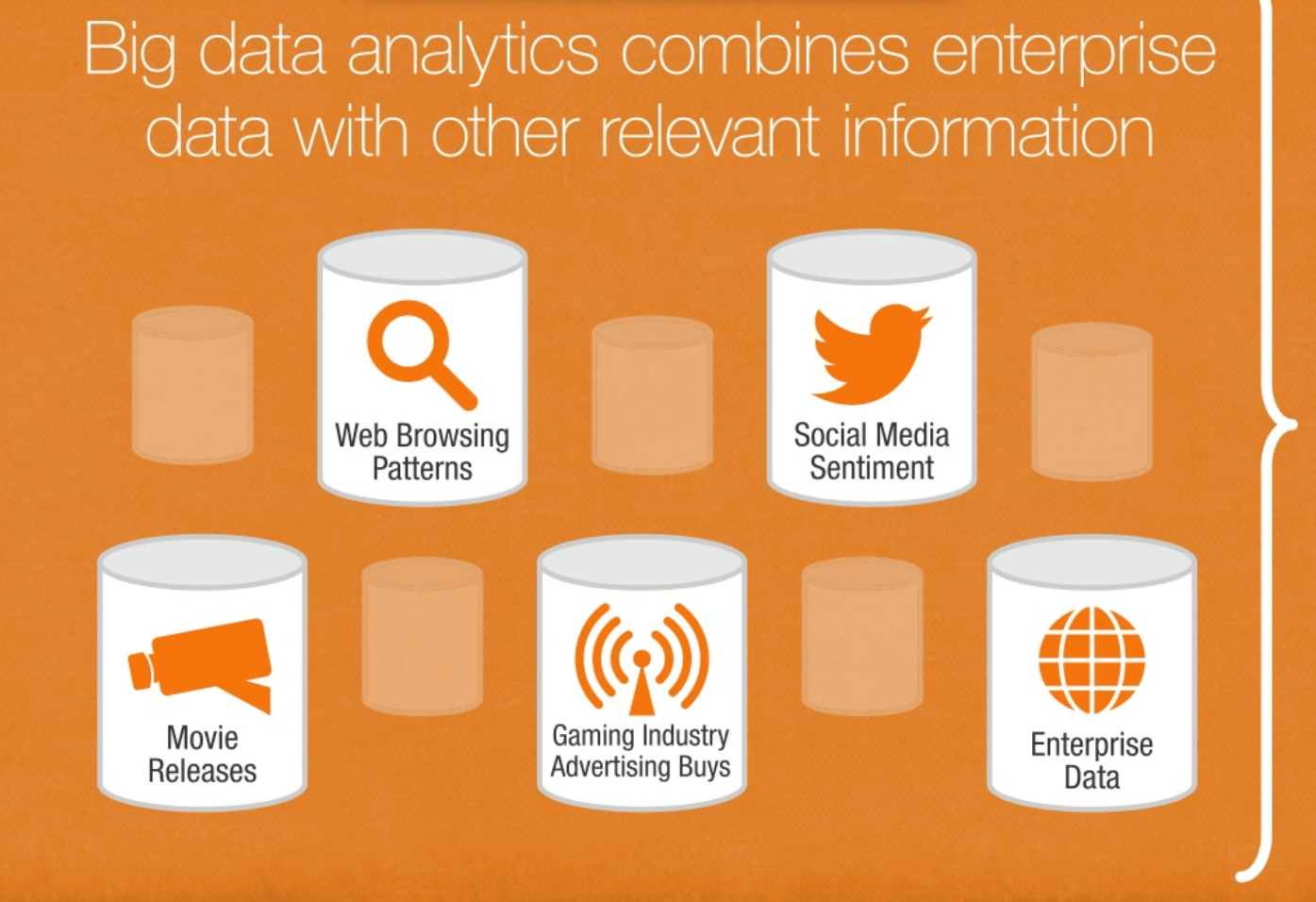
Big Data in Retail: Examples in Action.
How is all this relevant to today’s businesses? There are three specific ways in which big data can lead to better products and a better user experience—when viewed with context:
- Big data allows us to expand our knowledge of the customer and develop products and services that are best suited to their needs.
- Big data gives us a deeper understanding of how our customers behave, allowing us to connect with customers on a more meaningful level.
- Big data can help boost marketing activities, since it provides us with a chance to analyze customer behavior on multiple channels and understand when the customer is most likely to buy products or services.
In this article we’ll take a look at how to make the most of analytics, and why context is so important for big data.
Contextual data
A few years ago, one of my customers at UsabilityTools used our Form Tester tool to learn how users behave on his website. The Form Tester tool allows us to analyze the way website visitors interact with online forms. It provides data about each field of the form, identifying steps that cause dropouts.
The first thing that we noticed (thanks to the tool) was a high bounce rate, which indicated that something might be off with the form. In order to analyze the problem, we started to look at different elements of the form. We noticed that the usual response time was about 5 seconds, but one particular field took users over three minutes to fill. Having found a problem, we put it into the context: the field required an ID card number, which meant the user had to leave his desk, look for his wallet and come back to copy the data from the card. That explained the three minute wait, and showed us that we didn’t need to fix a problem with the form, so much as prepare users for needing their credit cards, so that they wouldn’t leave when they encountered this more “strenuous” task.
Contextual analytics is what allows businesses to trace patterns and detect trends like we did. It helps designers to build predictive models and prepare a suitable business strategy. The context is what makes the difference between “big data” and “dumb data.”
What’s wrong with no context?
Most companies in the digital industry already have some web analytics software implemented. But that doesn’t allow them to fully understand the psychological and cultural factors that influence customer lifestyles.
From the perspective of a business, the typical elements of web analytics, such as page views or the bounce rate, which provide data can actually lead to conclusions and mission-critical insights that are simply wrong. It’s easy to see how plain numbers can lie, especially when taken out of their context. Let’s take the “average time on site” metric. Five minutes as the average time looks pretty solid as an average, but when we look at individual visits, we suddenly see that the majority of visitors spend only ten seconds on the site, and the average metric is distorted by a few prolonged visits!
The realization that we can’t blindly trust data has been circulating for a while now – in an article entitled “What Data Can’t Do,” David Brooks of The New York Times points out that the main problem of big data is that it’s “pretty bad at narrative and emergent thinking, and it cannot match the explanatory suppleness of even a mediocre novel.” The best way to deal with this problem is to follow the words of Scott Gnau of Teradata Labs: “big data is a new piece, but it is not the only piece of the data puzzle.” Context and context-derived analytics can unlock the potential stored within big data; by contextualizing the data at hand, we can do things like improve our customer insights and identify the reasons behind common consumer behaviors. From here, businesses can create experiences that actually surprise and delight their users.
House of Cards is a popular political drama featured on Netflix, and perhaps one of the best examples of big data’s influence. Netflix actually uses big data to customize the House of Cards plotline and character twists. As Salon reported, if a user is watching the first episode and pauses it to get a snack, Netflix records the pause and the play. It’s impossible for Netflix to determine the reason why viewers paused the episode, but they can ask and assume – why do people pause at that moment? Is it because it’s shocking, repulsive, captivating or simply boring? Why do so many people rewind to exactly fourteen minutes into the episode? Is it because something is difficult to understand, or is it because the scene was amazing? Finally, why do viewers stopped watching the episode half-way through? The reason could be simple: the show was just bad.
By looking at the scenes during which these events (pause, rewind, stop) happen, the analytics team puts the events in context, and the results of their analysis are used later in order to improve the future viewing experience. Currently, according to rottentomatoes.com both seasons of House of Cards have received ratings well above 80%—proof that the series is successful. By putting big data in context, Netflix has prepared, and will improve a show which kept people glued to the screens.
House of Cards is an extreme example, but the same principle applies to any experience. Online delivery app Foodler recommends “best bets” to users based on previous items they have ordered from similar restaurants. They could go a step further and analyze their data within the context of time of day, and begin recommending breakfast-, lunch-, or dinner-specific foods at the appropriate times. Similarly, Target uses contextual big data to identify changes in customer behavior—this is how Target famously learned that a customer was pregnant before she had even told her family.
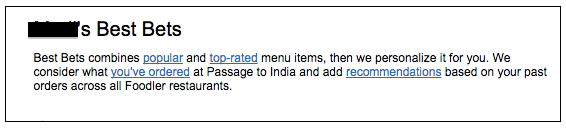
Foodler is able to predict what a user is likely to eat at any restaurant.
Knowing the why behind the data is what is really valuable. This context explains the psychology behind consumer behavior and consequently influences our ability to develop marketing strategies that successfully reach users at key touch points.
The Role of Context in Prediction
There is every reason to think that getting the hang of accurate models and patterns is key to boosting the analytics decision-making processes in the big data environment. Contextual analytics feed predictive analytics and produce a perspective on how people actually behave, crucial to building great predictive models.
We can use contextual analytics to emphasize data usability and business relevance. This allows us to create models that predict the future behavior of consumers, such as when Amazon recommends additional products.
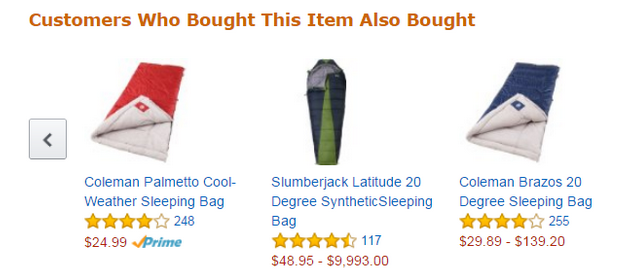
when I purchase a tent, Amazon uses analytics to determine I might want a sleeping bag as well.
Contextual analytics can determine, for instance, whether different data observations can be subscribed to a single individual, providing the context for the accurate merging of data to form real associations. For example, an e-commerce owner using predictive analytics will note that many customers purchase shoes on Friday afternoons, but contextual analytics will allow them to see that most of these customers are in office buildings, and are more likely to purchase when waiting for a client or meeting (in the last five minutes and first five minutes of the hour).
Data driven context | Possible actions |
---|---|
Repetitive consumer habits (e.g. buying a certain type of product or purchasing at certain times) | Suggest certain types of products or display offers during the purchase. |
Other consumer habits (e.g. products purchased by people with similar habits/demographics) | Suggesting products that other consumers picked |
Context derived from outside data (e.g. determining user’s hobbies based on their mobile apps) | Suggesting products concerning trending topics/people (e.g. recently awarded movies, deceased musicians) |
In a study of contextual analytics, Lisa Sokol and Steve Chen of IBM created another example, involving the traditional scoring system banks use to determine whether a client is eligible for a loan. If the bank uses analytics, they stated, it will see every account from every bank, but it won’t be able to associate all the different accounts from several banks to one person and, in consequence, will have to base its decision on imprecise information. With contextual analytics, on the other hand, the bank is able to see that those several accounts belong to one person and so will have all the necessary information to accurately evaluate the client’s ability to pay back a loan.
By taking advantage of context-driven analytics, we can increase the efficiency of prediction models and make better business decisions as a result.
Next Steps
Recognizing the benefits of using context in big data analytics is only the first step. Once we start gathering it, we’re ready to look for contextual insights and, as a result, create better customer experiences. Here are a few ways to get started.
- Study the data and KPIs that others are using, to better understand which are most relevant to the field.
- Don’t trust average values! Consider the context, whatever the metric is.
- Read more about the contextual analysis of big data:
- Age of Context: Mobile, Sensors, Data and the Future of Privacy by Robert Scoble
- The Human Face of Big Data by Rick Smolan
- Data Crush: How the Information Tidal Wave is Driving New Business Opportunities by Christopher Surdak
- Big Data Geeks blog
- Follow influencers on Twitter who often post useful articles on big data, such as Bob Gourley, Tony Baer or DJ Patil.
Analytics is more than just a numbers game. It's a way of tracking and analyzing user behavior over time. In this article, we explore this intersection of user experience and data, so that budding designers can add productive web analytics to their process.